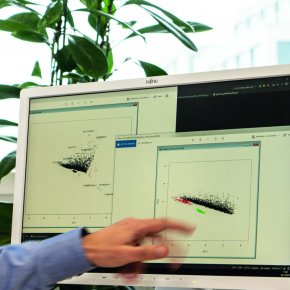
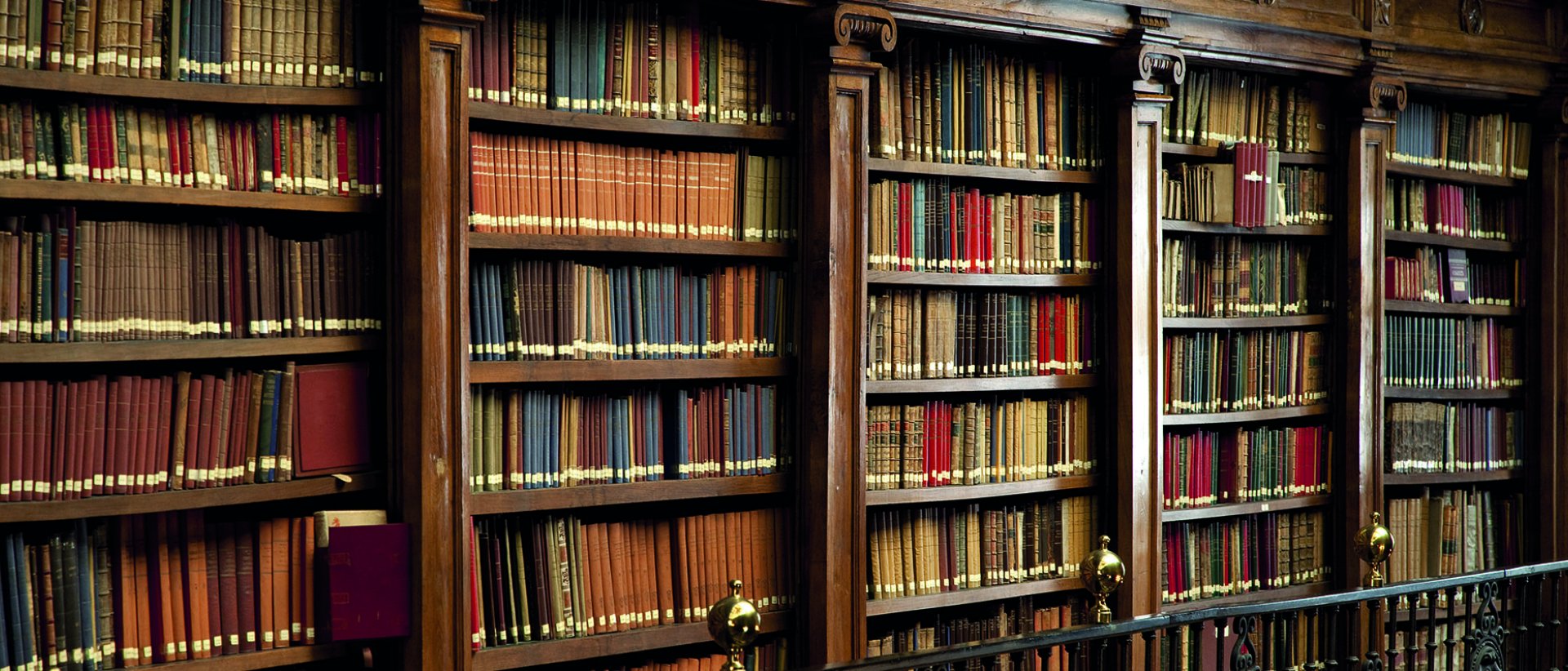
Data Analytics for Audi
Efficient library functions
From pattern recognition to automated error analysis
Cognizant Mobility supports Audi in the field of data science with its know-how, which has grown from experience across the automotive industry. This article reveals how your idea will also benefit from the help of Cognizant Mobility vision.
Identifying misconduct in complex IT systems is a tedious task.
As drivers and users of mobility services, we trust that no vehicle will reach the market without having completely eliminated its faults. And this is precisely the challenge, because when a fault occurs, it is important to find out which of the thousands of sensor data and conditions were responsible.
If one now considers that in reality all data and variables can occur in any combination, it becomes clear what difficulties the software developers are faced with. Finding patterns and anomalies is like searching in a haystack.
As an experienced data engineer, one knows that powerful analysis frameworks like Apache Spark can be used for such tasks. The application of such frameworks on a daily basis, across many departmental boundaries, presented Audi with a major problem, because time series analyses are time consuming and the handling of data is often manual. The CognizantMobility IT experts led by Angelique Jakob found the solution by developing a tool specifically for this purpose.
How the automated error analysis through Big Data Analytics works
It was possible to combine the best practices from Apache Spark into intelligent functions for the recurring use cases at Audi. With the help of these easy-to-use library functions, it is possible to search for patterns and events in large amounts of data across departments with little effort. Whether local maxima, rising/falling edges or other examples of anomalies in the time series - they can be found much easier and their effects on other variables can be traced.
As elegant as these library functions currently are, their future development will be exciting. This is because the Cognizant Mobility Team is already working internally on solutions for the complete automation of error analysis. Without human input, similarities in the signals and variables are to be combined into groups with the aid of various clustering methods. In this way, the software in the car can actually be enabled to fix itself.
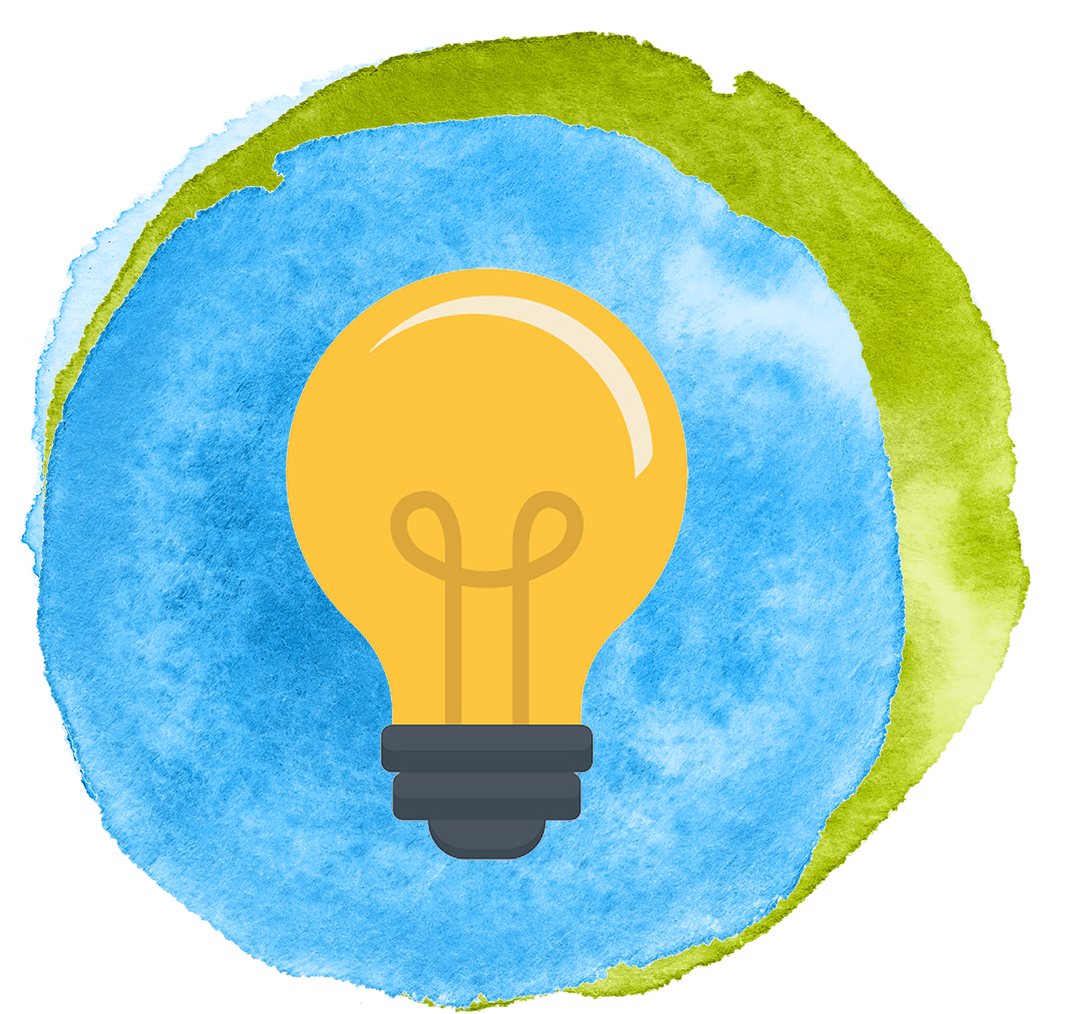
In A Nutshell
- Pattern Recognition
- Time series analysis
- Cluster procedure
- Apache Spark
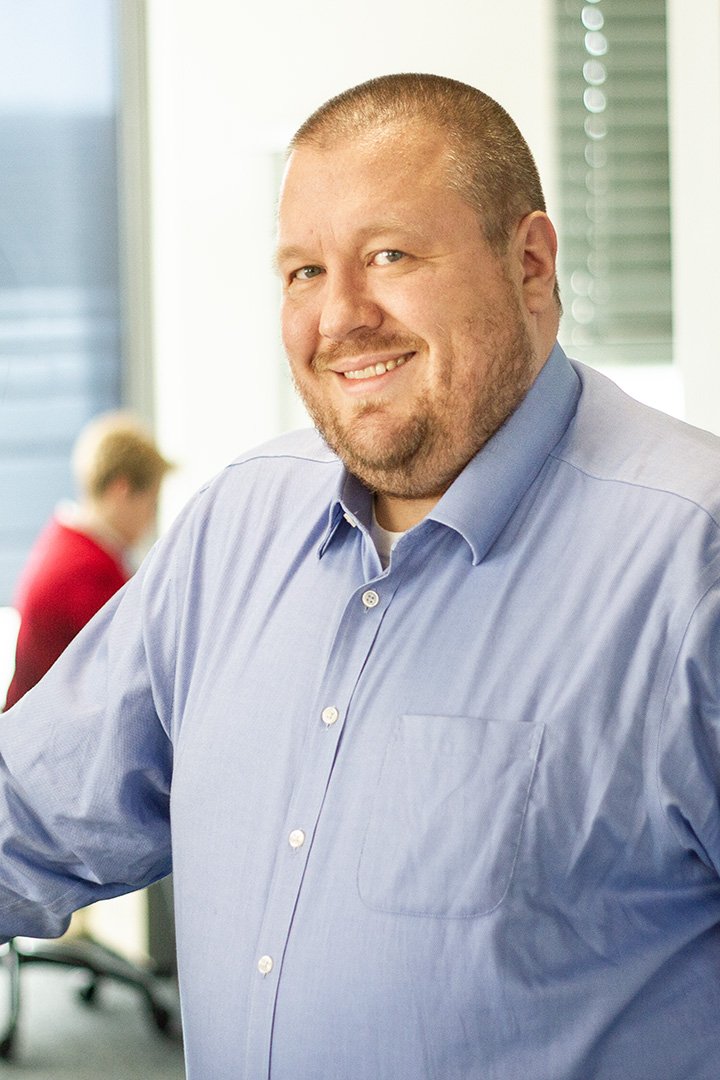